- Memorytamer 1 5 1 – Automatic Memory Freeing Application Status
- Memorytamer 1 5 1 – Automatic Memory Freeing Application Form
- Memorytamer 1 5 1 – Automatic Memory Freeing Application Requirements
- Memorytamer 1 5 1 – Automatic Memory Freeing Application Tool
- Memorytamer 1 5 1 – Automatic Memory Freeing Application Software
Whether it's your Windows, or smartphone, or any other device, RAM (Random Access Memory) plays a crucial role. So, how can you pick one of the best RAM Cleaner apps from the web? What factors do you usually consider when you're looking for a RAM booster for Windows 10? Can a RAM booster app optimize your PC's performance?
If your application is leaking then without a limit it will crash around 1.2 - 1.6 Gb (if memory serves). So 1 Gb is sensible. If during normal operation your application consumes not more than 100 Mb and you have many app pools on the server, than you should set the limit lower to prevent one app from damaging other apps. MemoryTameris an automatic memory-freeing app that runs in your menu bar. It supports notifications with both Growl and Notification Center, and also lets you free memory whenever you want with the 'Free memory now' menu item. On OS X 10.9, it gets even better! Wise Memory Optimizer is freeware, Any user can download it for free and enjoy free automatic update and technical support by email. It has been developed and fully tested to work great on Windows 10 and other Windows operating systems (both 64 and 32-bit), from Windows XP and up. No matter what you own - a desktop or a laptop. 5 Best RAM Cleaner and Booster For Windows 10 PC (Free/Paid) Let's get started and find out about the best RAM cleaner for PC. For our users, we have curated the list of the top 5 Windows 10 RAM cleaners software. You can take advantage of this and select the one which suits you best. Here is the list of 5 best RAM cleaners for PC in 2020. Click the Memory tab and check whether Free is near to Zero or is Zero. If it is, open RAMMap, click Empty on the Menu bar and Empty Standby list. This action instantly restores Free memory. The procedure using RAMMap is an effective workaround but the true solution lies in discovering the source of the problem.
Here's a summarized list of the 5 best RAM cleaners for Windows 10 to speed up your PC's performance.
List of Contents
- Why is RAM Important?
5 Best RAM Cleaner and Booster For Windows 10 PC (Free/Paid)
Let's get started and find out about the best RAM cleaner for PC. For our users, we have curated the list of the top 5 Windows 10 RAM cleaners software. You can take advantage of this and select the one which suits you best.
Here is the list of 5 best RAM cleaners for PC in 2020.
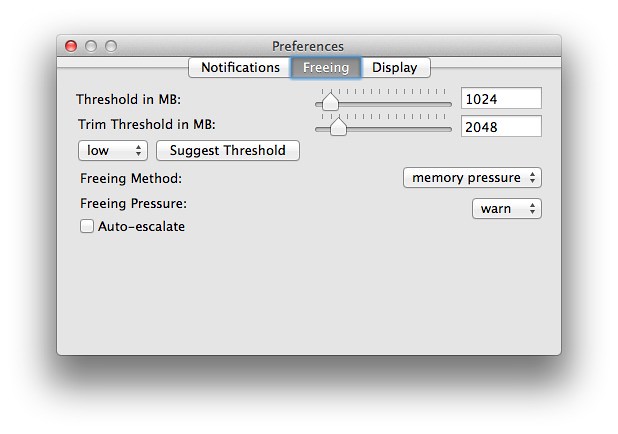
1. Advanced PC Cleanup
Advanced PC Cleanup is one of the best PC cleaner tool and optimize your PC. This tool comes with useful features to help you in improving the speed and performance of your computer. This will show you the system status along with the unwanted items and how much of disk space it hogs. This can be cleaned with the one- click solution and provide you with results in a few minutes. You can use the cleaning tools individually as well to remove junk, temporary files and trash from Recycle bin. Moreover, you can secure your system and data from the malicious content with the help of Advanced PC Cleanup. Uninstall as many applications you want to remove from the tool itself making it user friendly. Here are a few more features from Advanced PC Cleanup which makes it the best RAM cleaner for Windows 10.
Features
- Clears all invalid registry entries.
- Clean trash from Recycle bin.
- Removes the junk and redundant files.
- Uninstalls applications which are unused.
- Manages startup programs.
- Protects from malware.
2.Ashampoo WinOptimizer
Ashampoo WinOptimizer can not help you to optimize your system's performance but also make it more secure. This nifty RAM booster tool offers much-needed maintenance for your system and fine-tunes your PC's performance in a few clicks. Ashampoo WinOptimizer is one the best PC RAM cleaner because it quickly scans your entire Windows machine and clears the broken registry, gets rid of temp and junk files, and optimizes your PC's speed. Here are a few key highlights of the Windows 10 RAM cleaner tool:
Features
- Cleans the start-up folder of your system, resulting in faster boot time of your Windows PC or laptop.
- It helps you with eliminating browsing traces from all the web browsers you use, including Google Chrome, Safari, Edge, Opera.
- Whether you're a beginner or expert, Ashampoo WinOptimizer offers system maintenance to users of every skill level.
- Offers a deep cleaning of your RAM, allowing you to analyze your system's performance.
- The Internet Tuner feature of the tool helps you optimize network settings.
3. Advanced System Optimizer
Advanced System Optimizer is undoubtedly one of the best RAM cleaner tools available online. It offers overall system maintenance and protection to make your device new again. Advanced System Optimizer is easy to use clean up, and optimization tool for Windows power-packed with a bunch of useful features fit for your Windows optimization needs. Let's explore a few key highlights of this nifty RAM booster tool:
Features
- Cleans your system, including temp files, junk files, and all unwanted files that clutter your device's storage space.
- Defragments your PC's hard drive for better allocation of data, optimally utilizing system resources.
- It helps you in deleting and managing browsing history and cookies to keep your online browsing activities safe and secure.
- Instantly boosts up your PC's speed while freeing up RAM's storage space.
- Offers a dedicated Game Optimizer mode where you can enjoy a distraction-free gaming experience.
- It allows you to take a quick backup of system files, along with recovery options to retrieve data in case you accidentally delete any files.
4. Wise Memory Optimizer
Next on our list of best RAM cleaner for Windows 10 comes the Wise Memory Optimizer tool. Wise Optimizer tool is an affordable solution to clean up RAM on your Windows 10 system. This RAM cleaner for Windows 10 comes with a neat, easy to use interface, making the process of RAM cleaning and optimization a breeze. Let's learn a little more about Wise Memory Optimizer by taking a look at this RAM optimizer for Windows 10 features.
Features
- It offers automatic tune-up and optimization of RAM, where the optimization process can take place in the background even when the CPU is in an idle state.
- Rapidly finishes the scanning and optimizes your system's speed and performance.
- Compatible with almost all Windows versions and also for both 32 and 64-bit operating systems.
5. RAMRush
RAMRush can act as one of the best software to clean and boost RAM on your Windows PC. One of the best RAM cleaner for PC, it optimizes your system's performance while freeing up the physical RAM of your device, allowing your machine to run smoothly without any lags. Below mentioned are a few main features offered by the RAMRush cleaner and optimizer tool:
Memorytamer 1 5 1 – Automatic Memory Freeing Application Status
Features
- Easy to use, ideal for both beginners and experts.
- Boost your system's performance.
- It helps you in preventing memory leaks and system crashes.
- Displays real-time usage of RAM and CPU for better analysis.
- Offers Command-Line support.
Well, in this post, we will clear all your doubts and queries related to the RAM optimizer for Windows 10. But before that, let's get a quick understanding of why RAM is important for any machine.
Why is RAM Important?
Whenever you plan on buying any new device, say a smartphone or a laptop, you're always curious about RAM's storage space, right? RAM acts as the primary memory for any machine and it stores all sorts of important files and data which the CPU can directly access. As soon as your RAM's storage space gradually starts filling up app data and files, our device starts responding slowly.
This is when a RAM optimizer or RAM booster comes into the picture. The sole job of a RAM cleaner tool is to clean up your RAM's storage space so that your system becomes lightning fast again. To get into technical jargon, a RAM cleaner tool basically shifts all the processes and files to a virtual RAM so that there's less load on the physical RAM. Doing so keeps your RAM's space freed up so that your machine can stay optimized.
Conclusion
Here were some of the best RAM Cleaner tools for Windows 10 to optimize your PC's performance in just a few clicks. You can pick any RAM optimizer tool from the above-mentioned list to enjoy an optimal user experience on Windows.
And yes, before you leave don't forget to tell us which one is your favorite RAM booster tool for Windows? Feel free to hit the comments box.
Frequently asked questions-
Q1. How do I free up RAM on Windows 10?
Freeing up on Windows 10 is not a difficult task when you can use one of the RAM optimizers for Windows 10. Here we have given you the best of the options available to make the task easier for you by using the best PC RAM cleaner.
Q2. What is the best RAM cleaner for PC?
When it comes to the best RAM cleaner for PC, Advanced PC Cleanup does the job amazingly with its multiple tools for optimum performance. The overall system cleaning along with the memory management helps the PC free up space.
Q3. How much RAM can Windows 10 utilize?
When it comes to Windows 10 home edition 32 bit operating systems have the capacity of 4GB of RAM and 128GB for 64 bit operating systems. While this increases for the Windows
10 Pro edition, in usual, a 1GB to 3.5GB of RAM is used for the general work for a user.
Q4. How can I clean my computer RAM?
To clean the computer RAM, you can use multiple techniques. Uninstall the unwanted programs, clean the temporary and cache file on the system. Another option is to use one of the best RAM cleaners for PC to save you time as it analyzes the RAM on your computer.
Q5. Is there a free memory cleaner for Windows 10?
Yes, there are free memory cleaners available for Windows 10 just Advanced System Optimizer allows you to use it for free. It is allows you to get an optimized PC as it gives you more accurate results with secure features.
Q6. Istatistica 1 1 download free. What is the best RAM cleaner for my computer?
The best RAM cleaner for your computer can be the one that gives you the best results. In this case, we have given you the list of the best RAM cleaner for PC. You can select the one according to your requirements. We recommend you to use Advanced PC Cleanup as it tops the chart with the most features.
Q7. Why add more RAM in Windows 10?
Adding more RAM to your computer can help it to perform better. With the additional RAM, it is possible to open up more applications at the same time, your functions can be taken forward at the same time.
Q8. What software can I use to clean Windows 10?
You can use any of the above RAM cleaners for Windows 10. We recommend using Advanced PC Cleaner as it comes with a dedicated memory optimizer. It also gives the user a bunch of options to clear RAM when it exceeds a set limit. You can also set an automatic memory optimization for your computer.
Also, do not forget to like us & subscribe to our Social Media Channels!
Autoscaling is the process of dynamically allocating resources to match performance requirements. As the volume of work grows, an application may need additional resources to maintain the desired performance levels and satisfy service-level agreements (SLAs). As demand slackens and the additional resources are no longer needed, they can be de-allocated to minimize costs.
Autoscaling takes advantage of the elasticity of cloud-hosted environments while easing management overhead. It reduces the need for an operator to continually monitor the performance of a system and make decisions about adding or removing resources.
There are two main ways that an application can scale:
Vertical scaling, also called scaling up and down, means changing the capacity of a resource. For example, you could move an application to a larger VM size. Vertical scaling often requires making the system temporarily unavailable while it is being redeployed. Therefore, it's less common to automate vertical scaling.
Horizontal scaling, also called scaling out and in, means adding or removing instances of a resource. The application continues running without interruption as new resources are provisioned. When the provisioning process is complete, the solution is deployed on these additional resources. If demand drops, the additional resources can be shut down cleanly and deallocated.
Memorytamer 1 5 1 – Automatic Memory Freeing Application Form
Many cloud-based systems, including Microsoft Azure, support automatic horizontal scaling. The rest of this article focuses on horizontal scaling.
Note
Autoscaling mostly applies to compute resources. While it's possible to horizontally scale a database or message queue, this usually involves data partitioning, which is generally not automated.
Overview
An autoscaling strategy typically involves the following pieces:
- Instrumentation and monitoring systems at the application, service, and infrastructure levels. These systems capture key metrics, such as response times, queue lengths, CPU utilization, and memory usage.
- Decision-making logic that evaluates these metrics against predefined thresholds or schedules, and decides whether to scale.
- Components that scale the system.
- Testing, monitoring, and tuning of the autoscaling strategy to ensure that it functions as expected.
Azure provides built-in autoscaling mechanisms that address common scenarios. If a particular service or technology does not have built-in autoscaling functionality, or if you have specific autoscaling requirements beyond its capabilities, you might consider a custom implementation. A custom implementation would collect operational and system metrics, analyze the metrics, and then scale resources accordingly.
Configure autoscaling for an Azure solution
Azure provides built-in autoscaling for most compute options.
Azure Virtual Machines autoscale via virtual machine scale sets, which manage a set of Azure virtual machines as a group. See How to use automatic scaling and virtual machine scale sets.
Service Fabric also supports autoscaling through virtual machine scale sets. Every node type in a Service Fabric cluster is set up as a separate virtual machine scale set. That way, each node type can be scaled in or out independently. See Scale a Service Fabric cluster in or out using autoscale rules.
Azure App Service has built-in autoscaling. Autoscale settings apply to all of the apps within an App Service. See Scale instance count manually or automatically.
Azure Cloud Services has built-in autoscaling at the role level. See How to configure auto scaling for a Cloud Service in the portal.
These compute options all use Azure Monitor autoscale to provide a common set of autoscaling functionality.
- Azure Functions differs from the previous compute options, because you don't need to configure any autoscale rules. Instead, Azure Functions automatically allocates compute power when your code is running, scaling out as necessary to handle load. For more information, see Choose the correct hosting plan for Azure Functions.
Finally, a custom autoscaling solution can sometimes be useful. For example, you could use Azure diagnostics and application-based metrics, along with custom code to monitor and export the application metrics. Then you could define custom rules based on these metrics, and use Resource Manager REST APIs to trigger autoscaling. However, a custom solution is not simple to implement, and should be considered only if none of the previous approaches can fulfill your requirements.
Use the built-in autoscaling features of the platform, if they meet your requirements. If not, carefully consider whether you really need more complex scaling features. Examples of additional requirements may include more granularity of control, different ways to detect trigger events for scaling, scaling across subscriptions, and scaling other types of resources.
Use Azure Monitor autoscale
Azure Monitor autoscale provide a common set of autoscaling functionality for virtual machine scale sets, Azure App Service, and Azure Cloud Service. Scaling can be performed on a schedule, or based on a runtime metric, such as CPU or memory usage.
Examples:
- Scale out to 10 instances on weekdays, and scale in to 4 instances on Saturday and Sunday.
- Scale out by one instance if average CPU usage is above 70%, and scale in by one instance if CPU usage falls below 50%.
- Scale out by one instance if the number of messages in a queue exceeds a certain threshold.
Scale up the resource when load increases to ensure availability. Similarly, at times of low usage, scale down, so you can optimize cost. Always use a scale-out and scale-in rule combination. Otherwise, the autoscaling takes place only in one direction until it reaches the threshold (maximum or minimum instance counts) set in the profile.
Select a default instance count that's safe for your workload. It's scaled based on that value if maximum or minimum instance counts are not set.
For a list of built-in metrics, see Azure Monitor autoscaling common metrics. You can also implement custom metrics by using Application Insights.
You can configure autoscaling by using PowerShell, the Azure CLI, an Azure Resource Manager template, or the Azure portal. For more detailed control, use the Azure Resource Manager REST API. The Azure Monitoring Service Management Library and the Microsoft Insights Library (in preview) are SDKs that allow collecting metrics from different resources, and perform autoscaling by making use of the REST APIs. For resources where Azure Resource Manager support isn't available, or if you are using Azure Cloud Services, the Service Management REST API can be used for autoscaling. In all other cases, use Azure Resource Manager.
Consider the following points when using Azure autoscale:
Consider whether you can predict the load on the application accurately enough to use scheduled autoscaling, adding and removing instances to meet anticipated peaks in demand. If this isn't possible, use reactive autoscaling based on runtime metrics, in order to handle unpredictable changes in demand. Typically, you can combine these approaches. For example, create a strategy that adds resources based on a schedule of the times when you know the application is busiest. This helps to ensure that capacity is available when required, without any delay from starting new instances. For each scheduled rule, define metrics that allow reactive autoscaling during that period to ensure that the application can handle sustained but unpredictable peaks in demand.
It's often difficult to understand the relationship between metrics and capacity requirements, especially when an application is initially deployed. Provision a little extra capacity at the beginning, and then monitor and tune the autoscaling rules to bring the capacity closer to the actual load.
Configure the autoscaling rules, and then monitor the performance of your application over time. Use the results of this monitoring to adjust the way in which the system scales if necessary. However, keep in mind that autoscaling is not an instantaneous process. It takes time to react to a metric such as average CPU utilization exceeding (or falling below) a specified threshold.
Autoscaling rules that use a detection mechanism based on a measured trigger attribute (such as CPU usage or queue length) use an aggregated value over time, rather than instantaneous values, to trigger an autoscaling action. By default, the aggregate is an average of the values. This prevents the system from reacting too quickly, or causing rapid oscillation. It also allows time for new instances that are automatically started to settle into running mode, preventing additional autoscaling actions from occurring while the new instances are starting up. For Azure Cloud Services and Azure Virtual Machines, the default period for the aggregation is 45 minutes, so it can take up to this period of time for the metric to trigger autoscaling in response to spikes in demand. You can change the aggregation period by using the SDK, but periods of less than 25 minutes may cause unpredictable results. For Web Apps, the averaging period is much shorter, allowing new instances to be available in about five minutes after a change to the average trigger measure.
Avoid flapping where scale-in and scale-out actions continually go back and forth. Suppose there are two instances, and upper limit is 80% CPU, lower limit is 60%. When the load is at 85%, another instance is added. After some time, the load decreases to 60%. Before scaling in, the autoscale service calculates the distribution of total load (of three instances) when an instance is removed, taking it to 90%. This means it would have to scale out again immediately. So, it skips scaling-in and you might never see the expected scaling results.
The flapping situation can be controlled by choosing an adequate margin between the scale-out and scale-in thresholds.
Manual scaling is reset by maximum and minimum number of instances used for autoscaling.If you manually update the instance count to a value higher or lower than the maximum value, the autoscale engine automatically scales back to the minimum (if lower) or the maximum (if higher). For example, you set the range between 3 and 6. If you have one running instance, the autoscale engine scales to three instances on its next run. Likewise, if you manually set the scale to eight instances, on the next run autoscale will scale it back to six instances on its next run. Manual scaling is temporary unless you reset the autoscale rules as well.
The autoscale engine processes only one profile at a time. If a condition is not met, then it checks for the next profile. Keep key metrics out of the default profile because that profile is checked last. Within a profile, you can have multiple rules. On scale-out, autoscale runs if any rule is met. On scale-in, autoscale require all rules to be met.
For details about how Azure Monitor scales, see Best practices for Autoscale.
Memorytamer 1 5 1 – Automatic Memory Freeing Application Requirements
If you configure autoscaling using the SDK rather than the portal, you can specify a more detailed schedule during which the rules are active. You can also create your own metrics and use them with or without any of the existing ones in your autoscaling rules. For example, you may wish to use alternative counters, such as the number of requests per second or the average memory availability, or use custom counters to measure specific business processes.
When autoscaling Service Fabric, the node types in your cluster are made of virtual machine scale sets at the back end, so you need to set up autoscale rules for each node type. Take into account the number of nodes that you must have before you set up autoscaling. The minimum number of nodes that you must have for the primary node type is driven by the reliability level you have chosen. For more information, see scale a Service Fabric cluster in or out using autoscale rules.
You can use the portal to link resources such as SQL Database instances and queues to a Cloud Service instance. This allows you to more easily access the separate manual and automatic scaling configuration options for each of the linked resources. For more information, see How to: Link a resource to a cloud service.
When you configure multiple policies and rules, they could conflict with each other. Autoscale uses the following conflict resolution rules to ensure that there is always a sufficient number of instances running:
- Scale-out operations always take precedence over scale-in operations.
- When scale-out operations conflict, the rule that initiates the largest increase in the number of instances takes precedence.
- When scale in operations conflict, the rule that initiates the smallest decrease in the number of instances takes precedence.
In an App Service Environment, any worker pool or front-end metrics can be used to define autoscale rules. For more information, see Autoscaling and App Service Environment.
Memorytamer 1 5 1 – Automatic Memory Freeing Application Tool
Application design considerations
Memorytamer 1 5 1 – Automatic Memory Freeing Application Software
Autoscaling isn't an instant solution. Simply adding resources to a system or running more instances of a process doesn't guarantee that the performance of the system will improve. Consider the following points when designing an autoscaling strategy:
The system must be designed to be horizontally scalable. Avoid making assumptions about instance affinity; do not design solutions that require that the code is always running in a specific instance of a process. When scaling a cloud service or web site horizontally, don't assume that a series of requests from the same source will always be routed to the same instance. For the same reason, design services to be stateless to avoid requiring a series of requests from an application to always be routed to the same instance of a service. When designing a service that reads messages from a queue and processes them, don't make any assumptions about which instance of the service handles a specific message. Autoscaling could start additional instances of a service as the queue length grows. The Competing Consumers pattern describes how to handle this scenario.
If the solution implements a long-running task, design this task to support both scaling out and scaling in. Without due care, such a task could prevent an instance of a process from being shut down cleanly when the system scales in, or it could lose data if the process is forcibly terminated. Ideally, refactor a long-running task and break up the processing that it performs into smaller, discrete chunks. The Pipes and Filters pattern provides an example of how you can achieve this.
Alternatively, you can implement a checkpoint mechanism that records state information about the task at regular intervals, and save this state in durable storage that can be accessed by any instance of the process running the task. In this way, if the process is shut down, the work that it was performing can be resumed from the last checkpoint by using another instance.
When background tasks run on separate compute instances, such as in worker roles of a cloud-services–hosted application, you may need to scale different parts of the application using different scaling policies. For example, you may need to deploy additional user interface (UI) compute instances without increasing the number of background compute instances, or the opposite of this. If you offer different levels of service (such as basic and premium service packages), you may need to scale out the compute resources for premium service packages more aggressively than those for basic service packages in order to meet SLAs.
Consider using the length of the queue over which UI and background compute instances communicate as a criterion for your autoscaling strategy. This is the best indicator of an imbalance or difference between the current load and the processing capacity of the background task.
If you base your autoscaling strategy on counters that measure business processes, such as the number of orders placed per hour or the average execution time of a complex transaction, ensure that you fully understand the relationship between the results from these types of counters and the actual compute capacity requirements. It may be necessary to scale more than one component or compute unit in response to changes in business process counters.
To prevent a system from attempting to scale out excessively, and to avoid the costs associated with running many thousands of instances, consider limiting the maximum number of instances that can be automatically added. Most autoscaling mechanisms allow you to specify the minimum and maximum number of instances for a rule. In addition, consider gracefully degrading the functionality that the system provides if the maximum number of instances have been deployed, and the system is still overloaded.
Keep in mind that autoscaling might not be the most appropriate mechanism to handle a sudden burst in workload. It takes time to provision and start new instances of a service or add resources to a system, and the peak demand may have passed by the time these additional resources have been made available. In this scenario, it may be better to throttle the service. For more information, see the Throttling pattern.
Conversely, if you do need the capacity to process all requests when the volume fluctuates rapidly, and cost isn't a major contributing factor, consider using an aggressive autoscaling strategy that starts additional instances more quickly. You can also use a scheduled policy that starts a sufficient number of instances to meet the maximum load before that load is expected.
The autoscaling mechanism should monitor the autoscaling process, and log the details of each autoscaling event (what triggered it, what resources were added or removed, and when). If you create a custom autoscaling mechanism, ensure that it incorporates this capability. Analyze the information to help measure the effectiveness of the autoscaling strategy, and tune it if necessary. You can tune both in the short term, as the usage patterns become more obvious, and over the long term, as the business expands or the requirements of the application evolve. If an application reaches the upper limit defined for autoscaling, the mechanism might also alert an operator who could manually start additional resources if necessary. Note that under these circumstances the operator may also be responsible for manually removing these resources after the workload eases.
Related patterns and guidance
The following patterns and guidance may also be relevant to your scenario when implementing autoscaling:
Throttling pattern. This pattern describes how an application can continue to function and meet SLAs when an increase in demand places an extreme load on resources. Throttling can be used with autoscaling to prevent a system from being overwhelmed while the system scales out.
Competing Consumers pattern. This pattern describes how to implement a pool of service instances that can handle messages from any application instance. Autoscaling can be used to start and stop service instances to match the anticipated workload. This approach enables a system to process multiple messages concurrently to optimize throughput, improve scalability and availability, and balance the workload.
Monitoring and diagnostics. Instrumentation and telemetry are vital for gathering the information that can drive the autoscaling process.